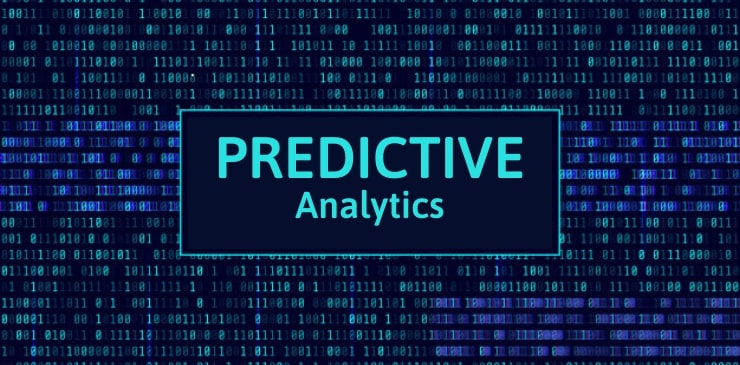
What Is Predictive Analytics?
Predictive Analytics is an umbrella term to refer to the set of processes that involve applying different computational techniques in order to make predictions about the future based on the available data, statistical algorithms and machine learning techniques.
In order to train the model to predict values, Predictive analysts apply known results, with different or completely new data, in a repetitive process.
Modelling provides results in the form of predictions represented by the degree of probability of the target variable based on the significance estimated from a set of input variables.
The Predictive Analytics considers what and why surrounding critical business problems, and provides calculated predictions of what a business might expect next. Predictive Analytics points to the future and is a bit more proactive with its findings.
Predictive analytics is used to detect business opportunities, detect and reduce fraud, customer retention, predict system failures. It is also used to detect cancer in patients, the evolution of epidemics, cost savings in organizations and speech recognition.
A Short History
Early statistical forecasting began in the 1950s with control charts and simple regressions run on mainframes. The 1980s saw commercial software such as SAS and SPSS bring predictive modelling to corporate desktops.
In 2006 the open-source library scikit-learn democratised algorithms for anyone with Python skills, while Hadoop let data scientists crunch terabytes. Cloud platforms released over the last decade removed most hardware barriers, so start-ups now wield the same horsepower once limited to global giants.
Building Blocks of a Predictive Analytics Workflow
1. Data Ingestion
Sensors, web logs, mobile apps, and point-of-sale systems spew raw facts around the clock. ELT pipelines capture streams into warehouses like Snowflake or lakehouses such as Delta Lake.
2. Data Preparation
Blank cells, typos, and duplicated rows poison forecasts. Cleaning scripts impute gaps, flag outliers, and enforce schema rules. Tokenisers split text, while parsers turn dates into ordinal numbers. Feature stores keep curated columns ready for real-time use.
3. Feature Engineering
Strong predictors rarely appear intact. Ratios, rolling aggregates, Fourier terms, holiday flags, word embeddings, and interaction effects often boost accuracy far more than exotic algorithms. Domain insight steers the search, yet automated tools like Featuretools scale discovery.
4. Model Selection and Training
A model is a function with tunable weights. Selecting one depends on latency budgets, data size, and interpretability needs. Training means minimising a loss function on a labelled set through optimisation methods such as gradient descent.
5. Validation
Hold-out sets estimate generalisation. K-fold cross validation splits data into equal parts, cycling through train and test slices. Time-series splits honour temporal order to avoid look-ahead bias.
Type | Metric Set | Practical Reading |
---|---|---|
Regression | RMSE, MAE, MAPE | Lower scores = tighter numeric forecasts |
Classification | Precision, Recall, F1, ROC AUC, PR AUC | Balance false alarms and misses |
Ranking | NDCG, MAP, Hit Rate | Higher scores = better ordered lists |
Survival | Concordance Index, Brier Score | Closer to ideal = closer to truth |
6. Deployment
Batch scoring runs overnight. Real-time endpoints return predictions in milliseconds through REST or gRPC. Edge deployment pushes compressed models to phones or embedded boards.
7. Monitoring and Retraining
Concept drift sneaks in after product launches. Dashboards track prediction error, data distribution, and latency. When drift crosses a guardrail, automated retraining pipelines re-fit the model and publish a fresh version.
Statistical Techniques Behind Predictive Analytics
- Linear Regression models the mean of a continuous target as a weighted sum of inputs plus noise.
- Logistic Regression estimates the log-odds of a binary outcome and remains popular in credit scoring.
- Poisson and Negative Binomial Regression handle count data such as call-centre arrivals.
- ARIMA and SARIMA explain time-series using autoregressive and moving-average terms plus seasonality.
- Survival Models including Cox Proportional Hazards predict time until an event, aiding churn prevention.
Machine Learning Techniques for Predictive Analytics
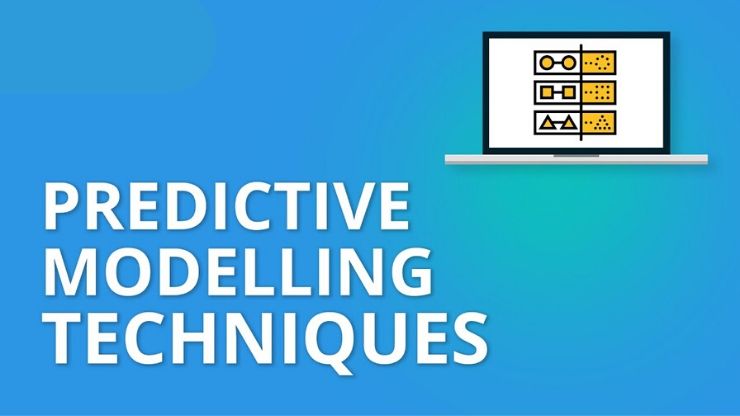
1. Decision Tree Family
A single CART tree is a flowchart of if-else splits. Random Forests create hundreds of trees on bootstrapped data and average their votes, reducing variance. Gradient Boosting—XGBoost, LightGBM, CatBoost—adds trees sequentially, each new learner focusing on residuals from the prior stage.
2. Support Vector Machines
SVMs seek the widest margin between classes. Kernel tricks let them draw non-linear boundaries while still solving a convex problem.
3. k-Nearest Neighbours
Predictions rely on the closest examples by Euclidean or cosine distance. Simplicity aids transparency yet memory and latency can grow with data size.
4. Neural Networks
Dense feed-forward networks approximate almost any function. Convolutional layers excel at images; recurrent cells read sequences; attention-based transformers now rival older designs on tabular tasks.
5. Probabilistic Methods and Bayesian Updating
Gaussian Processes deliver a confidence band along with a mean prediction. Bayesian Networks encode causal assumptions and update beliefs as fresh evidence arrives.
5. AutoML and Neural Architecture Search
AutoML platforms explore model families and hyper-parameters through Bayesian optimisation or evolutionary search, saving teams with limited staff hours.
Feature Selection Strategies
Irrelevant columns hurt accuracy and inflate compute bills.
- Filter Methods: Chi-square tests, mutual information, or Pearson correlation rank attributes before modelling.
- Wrapper Methods: Recursive Feature Elimination prunes the weakest features.
- Embedded Methods: Regularised algorithms such as Lasso shrink useless coefficients toward zero during training.
Popular Predictive Analytics Tools and Platforms
Category | Examples | Strengths |
---|---|---|
Open Source Libraries | scikit-learn, XGBoost, Prophet, Statsmodels | Free, transparent code, large community |
Big-Data Frameworks | Apache Spark ML, Flink ML | Distributed memory, petabyte scale |
Visual GUI Suites | KNIME, RapidMiner, IBM SPSS Modeler | Drag-and-drop, low-code |
Cloud Services | AWS SageMaker, Google Vertex AI, Azure ML | Managed pipelines, auto-scaling |
MLOps Platforms | MLflow, Kubeflow, BentoML | Versioning, model registry, experiment logs |
Industry Use Cases of Predictive Analytics
1. Banking and Insurance
Fraud scoring flags risky transactions before money leaves the vault. Underwriting models weigh applicant attributes to price policies within seconds.
2. Retail and E-Commerce
Demand forecasting aligns procurement with expected sales, trimming stock-outs and clearance markdowns. Recommender systems lift average order value by suggesting products often bought together.
3. Healthcare
Early warning systems monitor vitals and lab results, alerting staff to septic shock or respiratory failure. Genomic models predict drug response, guiding personalised medicine.
4. Manufacturing and Energy
Predictive maintenance on turbines, pumps, or conveyor belts removes unplanned downtime. Remaining useful life models extend equipment life and reduce spare-parts hoarding.
5. Telecommunications
Churn models estimate which subscribers might leave next month so retention teams can offer custom incentives.
6. Sports and Entertainment
Front offices analyse player tracking data to forecast performance peaks and optimise scouting budgets. Streaming platforms schedule fresh content drops based on projected viewer demand.
Ethics, Governance, and Regulation
Bias can creep in when historical data reflects past prejudice. Statistical parity checks, disparate impact tests, and counterfactual fairness scores reveal skewed outcomes backed by numbers.
Regulators pay close attention. The EU’s AI Act sets risk tiers and mandates transparency reports. GDPR Article 22 guards citizens against solely automated decisions. Validation documents, audit trails, and model cards provide evidence of due diligence.
Frequent Challenges and How to Tackle Them
Challenge | Symptoms | Mitigation Approach |
---|---|---|
Data Leakage | Test accuracy unusually high | Strict temporal splits, feature checklists |
Overfitting | Training error far below validation | Cross validation, regularisation, early stop |
Concept Drift | Rising error after deployment | Scheduled retrain, adaptive learning |
Imbalanced Classes | Rare positives drown signal | SMOTE, focal loss, cost-sensitive learning |
Latency Constraints | Predictions exceed SLA | Feature caching, model pruning, hardware accel |
Best Practices for Predictive Analytics Projects
- State a measurable objective such as “cut customer churn by two points in nine months.”
- Assemble a balanced team spanning domain experts, data engineers, scientists, developers, and an executive sponsor.
- Invest in data quality; no amount of modelling rescues dirty input.
- Document every assumption in README files inside the repository.
- Keep pipelines modular so models swap without rebuilding ingestion.
- Automate testing for data schema, code style, and prediction sanity.
- Establish feedback loops; push prediction outcomes back for continuous learning.
- Measure ROI by comparing lift against a randomised control group.
Emerging Trends Shaping Predictive Analytics
- Real-Time Stream Processing with Kafka and ksqlDB powers instant fraud stops.
- Edge Intelligence pushes models onto microcontrollers in smart locks or wearables.
- Graph Neural Networks reveal fraud rings or molecule properties better than flat features.
- Federated and Split Learning keeps data on-premises while sharing encrypted gradients.
- Synthetic Data Generation with GANs and diffusion models fills privacy or rarity gaps.
- Quantum-Inspired Optimisers tackle portfolio and routing problems on hybrid hardware.
Step-by-Step Implementation Roadmap
Phase | Key Actions | Deliverables |
---|---|---|
Discovery | Align with stakeholders, define KPI, audit data | Project charter, success metric |
Proof of Concept | Build sample pipeline, run baseline, estimate lift | POC report, cost–benefit estimate |
Production Build | Harden code, build CI/CD, set alert thresholds | Deployable artefact, monitoring dashboard |
Launch | Roll out in stages, run A/B test, gather feedback | Live predictions, uplift measurement |
Scale-Up | Add new data sources, retrain schedule, iterate | Versioned models, retrained performance |
Measuring Return on Investment
Many pilots stall when leaders fail to see bottom-line gains.
- Incremental Revenue = (Average order value after model − Baseline) × Number of orders.
- Cost Savings = (Failure rate before – Failure rate after) × Cost per failure.
- Model Operating Expense = Cloud compute + Licences + Headcount.
ROI = (Incremental Revenue + Cost Savings − Model Operating Expense) ÷ Model Operating Expense.
Hold out a fraction of traffic to observe outcomes with and without predictions, isolating the model effect from other campaign factors.
Hyper-parameter Tuning Methods
Parameters learned during training differ from hyper-parameters set before the run.
- Grid Search tests every combination in a defined range—exhaustive yet slow.
- Random Search samples uniformly, often finding strong settings faster.
- Bayesian Optimisation builds a surrogate of the objective function and explores promising points.
- Hyperband and Successive Halving allocate resources adaptively, pruning weak settings early.
- Evolutionary Algorithms mutate and cross-over candidate sets, mimicking natural selection.
Case Study: Airline Fuel Planning
A mid-size carrier struggled with rising fuel costs. Dispatchers used fixed fuel buffers regardless of weather or congestion, leading to over-carriage.
- Data: Three years of flight plans, actual burn, wind forecasts, and airport queue data.
- Model: Gradient Boosting Regressor predicted reserve fuel for each sector.
- Validation: Time-series split guarded against leakage; MAE tracked error.
- Result: Extra fuel loaded fell by 120 kg per flight. At $0.75 per kg, yearly savings hit $6.5 million, dwarfing $400 000 in cloud and staff cost.
- Lesson: Business alignment mattered more than algorithm novelty; clarity on economic value secured budget for phase two.
Cloud Cost Management Tips
- Schedule training jobs during off-peak hours when spot instances cost less.
- Store cold data on object storage, shifting only fresh partitions to fast disks.
- Use auto-scaling endpoints that spin down when traffic drops.
- Rights-size GPU clusters; many tabular tasks gain little from high-end GPUs.
- Cache features so batch jobs avoid regenerating heavy joins each run.
Testing and Quality Assurance
- Data Tests: Assert row counts, schema, and value ranges at ingestion.
- Model Tests: Verify expected shape of output and correlation with ground truth.
- Integration Tests: Deploy the pipeline to staging and simulate live calls.
- Shadow Mode: Run the new model alongside the old one without influencing decisions, comparing metrics in real time.
Cross-Industry Standard Process
Many firms adopt CRISP-DM, a vendor-neutral framework with six phases: Business Understanding, Data Understanding, Data Preparation, Modelling, Evaluation, and Deployment. The loop mindset guides sprint planning, ensuring projects do not rush from prototype to production without stakeholder sign-off.
Glossary of Key Terms
Term | Plain-English Meaning |
---|---|
Feature | A column used as input to a model |
Label | The target value the model aims to predict |
Overfitting | When a model memorises noise instead of learning signal |
Concept Drift | Change in the relationship between inputs and target |
Hyper-parameter | Setting chosen before training that shapes behaviour |
ROC Curve | Plot of true-positive rate against false-positive rate |
SHAP Values | Scores that explain how each feature shifts a prediction |
Feature Store | Managed repository of curated features for reuse |
Conclusion
Predictive Analytics offers a pragmatic way to peer around corners. When guided by clear goals and ethics, forecasts sharpen planning, cut waste, and unveil growth pockets.
Successful programmes pair clean pipelines and robust models with ongoing monitoring, human oversight, and a plan to adapt as the world changes. Teams mastering these habits place themselves a step ahead, ready to ride tomorrow’s waves rather than chase yesterday’s ripples.
Also Read: