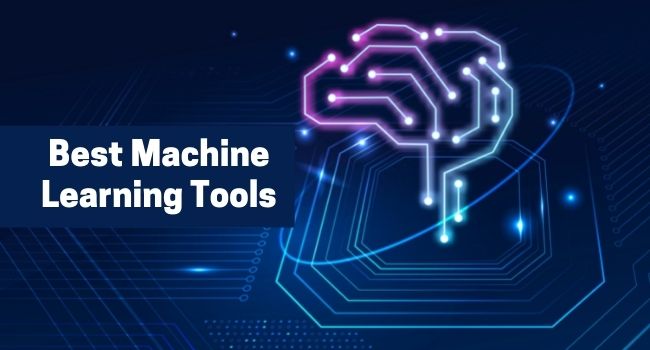
Machine learning now sits at the heart of fraud detection, drug discovery, and personal assistants. Choosing the right software decides project speed, cost, and quality.
Mastering machine learning tools will let you play with the data, train your models, discover new methods, and create your own algorithms.
So, why not think of starting a career in this exciting field. You don’t need to go to a university and follow an academic curriculum.
Machine learning comes with an extensive collection of ML tools, platforms, and software. Out of many machine learning tools, choose any one of them to gain expertise.
Here in this article, we will discuss the top 15 best and most popular machine learning tools. Let us get started without any ado!
Best Machine Learning Tools
1. TensorFlow
Backed by Google, TensorFlow supplies deep-learning libraries that scale from laptops to clusters. Graph execution, eager mode, and hardware acceleration form the core. JavaScript, Swift, and Rust bindings widen reach.
TFX pipeline components bring data validation, training, and model serving under one roof. An engineer can fine-tune a vision model on a Sunday and ship it in a container on Monday.
Stand-out features
- Auto-mixed precision for faster GPU training
- Keras high-level API simplifies layer stacking
- TensorBoard tracks metrics with one‐line logging
- SavedModel format supports cross-language inference
- TF-Lite covers on-device inference for phones and edge chips
- TF-Agents speeds reinforcement learning experiments
Pricing
- Open-source under Apache 2.0; zero license fee
- Cloud TPU v5p (preview) starts around $3.22/h; billing by Google Cloud
2. PyTorch
Born at Meta AI research, PyTorch wins hearts with dynamic computation graphs. Tensors act like NumPy arrays meshed with GPU power.
Debugging feels natural in any IDE, which draws academics and production teams alike. The torch.compile() function, introduced in 2.0, pushes speed to new highs while keeping code readable.
Key features
- Dynamic graph execution enables on-the-fly changes
- torchtext, torchvision, torchaudio handle common datasets
- DistributedDataParallel scales to thousands of GPUs
- Native AMP halves training time on many workloads
- Torch-Serve handles model, version, and scaling knobs
- Strong community; most new papers ship PyTorch code first
Pricing
- BSD-licensed; no cost for local use
- PyTorch on AWS DLAMI: pay only for EC2 compute from ≈$0.15/h (t4g.small)
3. Scikit-Learn
For tabular data, scikit-learn remains the Swiss army knife. Classification, regression, clustering, and preprocessing live behind a unified API. Pipelines reduce leakage risk, while model selection tools guide hyperparameter search. Tight integration with pandas turns raw CSVs into clean features in minutes.
Feature list
- Over 100 algorithms, from Random Forest to Gradient Boosting
- cross_val_score and GridSearchCV for fair evaluation
- ColumnTransformer joins numeric and text paths
- Joblib parallelism spreads CPU load
- Simple interoperability with ONNX for runtime exports
Pricing
- BSD-style license; free forever
- Optional commercial support from vendors such as Enthought, starting near $1,500 per seat / year
4. Keras
Keras rides atop TensorFlow yet sports its own slim surface. Layers line up like bricks, letting even newcomers craft CNNs or transformers without wrestling with tensors. The Functional API supports complex directed-acyclic graphs. Callbacks knit training with checkpoints, early stopping, and custom actions.
Features
- model.fit() one-liner for training loops
- Built-in image and text preprocessing layers
- tf.keras.mixed_precision for float16 gains
- Integration with TensorFlow Hub for plug-and-play models
- Auto-Keras extension offers AutoML flavor
Pricing
- Included with TensorFlow; open-source
- Enterprise support folded into Google Cloud premium plans
5. Amazon SageMaker
SageMaker bundles notebooks, feature store, experiment tracking, and managed endpoints. Engineers upload data to S3, choose a built-in container, and hit “Train.”
Automatic model tuning hunts best hyperparameters. Managed spot training drops bills by up to 90 %. The new SageMaker Canvas adds no-code prediction for analysts.
Highlights
- 200+ pre-built algorithms and images
- Model Monitor checks drift in real time
- Clarify audits bias and explains predictions
- Serverless inference scales to zero
- Feature Store keeps lineage across teams
- Pipelines automate CI/CD for ML
Pricing
- Studio notebooks: t3.medium at ≈$0.058/h
- ml.m5.xlarge training instance: ≈$0.25/h
- Data processed and storage charged separately
6. Google Vertex AI
Vertex AI weaves data, training, tuning, and serving into one GCP console. AutoML handles vision, tabular, and text tasks with drag-and-drop drives. Custom jobs support PyTorch and TensorFlow images. Feature Store, Matching Engine, and Model Registry shorten hand-offs between teams.
Features
- Workbench notebooks with one-click GPU
- Hyperparameter tuning powered by Vizier
- Predictions on CPUs, GPUs, or the latest TPU v5e
- Explainable AI delivers SHAP-style attributions
- Continuous evaluation against live traffic
- Native integration with BigQuery and Dataflow
Pricing
- Training on n1-standard-4 + 1 NVIDIA T4: ≈$0.77/h
- AutoML Tables node hour: ≈$2.80
- First 5 GB Feature Store storage free each month
7. Microsoft Azure Machine Learning
Azure ML combines low-code designer canvases with SDK-driven pipelines. Responsible AI dashboards flag fairness, privacy, and performance. Managed online endpoints bring blue-green traffic shifting. Integration with GitHub Actions streamlines CI/CD.
Notable items
- AutoML sweeps algorithms and preprocessing
- ML Assist suggests next steps in notebooks
- Managed Feature Store supports versioning
- Prompt Flow (2025 preview) tests LLM prompts at scale
- Secure enclaves for confidential inference
- Built-in monitoring hooks for OpenTelemetry
Price notes
- Basic tier free; pay-as-you-go for compute
- Standard D4 v5 compute: ≈$0.23/h
- AutoML experiment charge ~ $0.003 per node second
8. Databricks Lakehouse ML
Databricks fuses Spark data engineering with MLflow tracking. The Lakehouse architecture stores structured and unstructured bits in Delta tables, avoiding copies. Runtime for Machine Learning pre-installs popular libraries and GPU drivers.
Core points
- Auto-loader ingests streaming data without code
- Feature Engineering in notebooks or Delta Live Tables
- MLflow Experiment UI shows parameters vs. metrics
- Photon engine speeds SQL joins for feature prep
- Unity Catalog secures data and model permissions
Pricing
- Pay per DBU (Databricks Unit); jobs light cluster on AWS ≈$0.22/DBU
- Workspace edition adds serverless compute; price varies by region
9. RapidMiner
RapidMiner offers a visual flow for data prep, modeling, and validation. Over 1,500 drag-and-drop operators mean domain experts craft pipelines with no script. Python and R scripting nodes satisfy coders. The Turbo Prep view accelerates cleaning tasks.
Feature picks
- Auto-Model suggests best algorithms with bias checks
- Real-time scoring via REST
- Extensions for text mining and anomaly detection
- Built-in governance dashboard
- Collaboration through shared repositories
Cost
- Free tier: 10,000 rows per project
- Professional plan: ≈$7,500 / user / year
- Enterprise license quoted case-by-case
10. H2O Driverless AI
Driverless AI automates feature engineering with evolutionary search. Time-series lag creation, text vectorization, and target encoding appear without manual tweak. A model leaderboard ranks winners by accuracy, speed, and interpretability.
Features
- GPUs cut AutoML time to minutes
- MOJO models run in Java, C++, or Python runtimes
- Shapley-based explanations for each row and global shape
- AutoDoc exports PDF with methodology
- Smart re-training on new data only
Pricing
- Free trial for 21 days
- Announced list price: ≈$25,000 / CPU core / year, volume breaks available
11. IBM Watson Studio
Watson Studio sits on IBM Cloud or on-prem. JupyterLab, RStudio, and SPSS Modeler coexist in one workspace. AutoAI builds pipelines and deploys them to Watson Machine Learning.
Features
- Federated Learning trains where data lives
- Bias Monitor flags disparate impact
- Model drift alerts with retrain suggestions
- Visual SPSS flow for statisticians
- Git integration for version control
Pricing
- Lite plan: 10 capacity unit hours per month free
- Standard: $99 / month + usage overage; extra GPU at ≈$1.60/h
12. KNIME Analytics Platform
KNIME supplies a node-based desktop that chains readers, transformers, and learners. Community nodes plug in H2O, TensorFlow, even Snowflake connectors. The new Modern UI refresh keeps things snappy.
Features
- Local execution free with unlimited rows
- KNIME Hub hosts thousands of example workflows
- Integrated LLM nodes for GPT-4 prompting
- Server edition schedules jobs and manages access
- Hybrid data apps with interactive views
Price
- Desktop: free, open-source GPL
- KNIME Server Small: ≈$9,900 / year
13. DataRobot AI Platform
DataRobot targets business users who need guided automation. After CSV upload, DataRobot profiles data, tries dozens of blueprints, and surfaces champion models with plain-speech insights. Governed ML Ops watches models once they hit production.
Features
- Automated feature discovery and ranking
- Time series with automatic holiday detection
- What-if analysis dashboards
- Continuous Learning flag for each model
- Bias heatmaps for regulators
Pricing
- Starter SaaS: quote ≈$15,000 / year for up to 100 M rows
- Enterprise on-prem negotiable; multi-million annual typical
14. MLflow
MLflow tracks experiments, handles model packaging, and provides a registry. Widely adopted thanks to its lightweight design and REST API. Plugins extend support for TensorFlow, XGBoost, and Spark.
Features
- mlflow run executes reproducible projects
- Model registry stages: Staging, Production, Archived
- Supports Docker and Conda environments
- Metric comparison UI with parallel coordinate plots
- Works on local file store or SQL database
Pricing
- Open-source Apache 2.0
- Databricks managed MLflow billed per DBU as noted earlier
15. Apache Spark MLlib
Spark MLlib scales classic machine-learning over distributed data frames. Pipelines echo scikit-learn but run across clusters. ALS for recommendation, gradient-boosted trees for ranking, and streaming k-means for live segmentation come standard.
Features
- In-memory processing reduces disk I/O
- DataFrame-centric API in Scala, Java, Python, R
- One-hot, hashing, and word2vec transformers
- CrossValidator for grid search across nodes
- GraphX add-on for graph analytics
Cost
- Free under Apache 2.0
- Spark on AWS EMR m5.xlarge: ≈$0.192/h plus EMR $0.042/h surcharge
Conclusion
TensorFlow and PyTorch rule deep learning. Scikit-learn shines for structured data. Cloud suites—SageMaker, Vertex AI, and Azure ML – trade flexibility for hands-off scaling.
Visual builders KNIME and RapidMiner speed non-coders, while Driverless AI and DataRobot chase automation. MLflow, Spark MLlib, and Databricks handle lineage and scale for data engineers.
Pricing ranges from open-source freedom to five-figure enterprise bundles. Study team skill, budget, and regulatory needs before signing a purchase order.
With the shortlist above, any organization can match project goals to the right engine and push models from idea to inference without wandering in the dark.
Also Read:
1 comment
Hi,
Nice blog on machine learning tools, good knowledgeable tools. You have covered almost all tools and their uses.